Diyar Altinses, M.Sc.
FB Elektrische Energietechnik
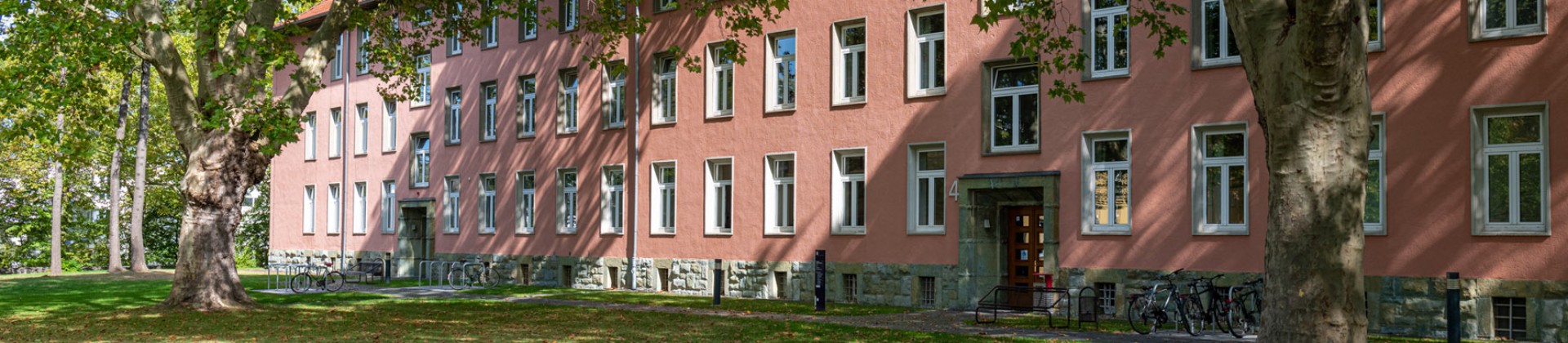
Zur Person
September 2019 - April 2021
Double Degree: Master of Science (M.Sc.)
South Westphalia University of Applied Sciences, Soest (Germany)
University of Bolton, Bolton (UK)
Course: Systems Engineering and Engineering Management
since November 2021
Degree: Doctor of natural sciences (Dr. rer. nat.)
Graduate School for Applied Research, North Rhine-Westphalia (Germany)
South Westphalia University of Applied Sciences, Soest (Germany)
Title: Advancing Multimodal Fault-tolerance: Leveraging Intermodal and Intramodal Correlations using ML Algorithms
Forschung
Optimization of industrial processes has become increasingly important since unplanned downtime in large industries is associated with increasingly higher costs. These costs come from production lines with higher capacity and higher spending on energy, labor, and material wastage. Predictive maintenance plays a critical role in reducing costs and increasing productivity by predicting wear and aging processes. Nevertheless, predictive maintenance can only detect predictable wear and aging processes. However, failures can also occur randomly and usually lead to unpredictable downtime. A promising and innovative approach to address failures and ensure robust operation is to apply fault-tolerant machine learning techniques to reconstruct corrupt sensor data. This Fault-tolerant systems comprise three integral components: fault injection, fault detection, and fault correction. In this research, we mainly focus on multimodal real-world failure injection methods and self-supervised, as well as generative corretion approaches. We do not specifically address fault detection, as the correction mechanism is consistently applied, rendering detection an inherent and integrated aspect of the correction process.
Projekte
Drones4Parcel5G: Entwicklung eines Systems zur Planung und Bewegungssteuerung von Lieferflügen mittels Paket-Drohnen auf Basis der 5G-Funktechnologie
PSAR: Entwicklung eines lernfähigen KI-gestützten, markerlosen AR-Assistenzsystems für unterschiedliche industriellen Bedienhandlungen von komplexen Systemen mit automatisierter AR-Inhalterstellung (Zeitersparnis > 90 %)
Publikationen
2023
D. Altinses and A. Schwung, "Deep Multimodal Fusion with Corrupted Spatio-Temporal Data Using Fuzzy Regularization," IECON 2023- 49th Annual Conference of the IEEE Industrial Electronics Society, Singapore, Singapore, 2023, pp. 1-7, doi: 10.1109/IECON51785.2023.10312522.
D. Altinses and A. Schwung, "Multimodal Synthetic Dataset Balancing: a Framework for Realistic and Balanced Training Data Generation in Industrial Settings," IECON 2023- 49th Annual Conference of the IEEE Industrial Electronics Society, Singapore, Singapore, 2023, pp. 1-7, doi: 10.1109/IECON51785.2023.10311948.